Core Hadoop ecosystem is nothing but the different components that are built on the Hadoop platform directly. However, there are a lot of complex interdependencies between these systems.
Before starting this Hadoop ecosystem tutorial, let’s see what we will be learning in this tutorial:
- What is Hadoop Ecosystem?
- HDFS
- YARN
- MapReduce
- Apache Pig
- Apache Hive
- Apache Ambari
- Mesos
- Apache Spark
- Tez
- Apache HBase
- Apache Storm
- Oozie
- ZooKeeper
- Data Ingestion
There are so many different ways in which you can organize these systems, and that is why you’ll see multiple images of the ecosystem all over the Internet. However, the graphical representation given below seems to be the best representation so far.
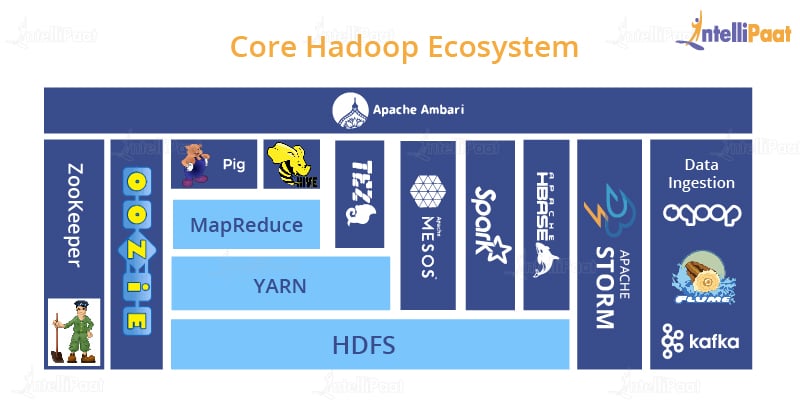
The light blue-colored boxes you see are part of Hadoop, and the rest of them are just add-on projects that have come out over time and integrated with Hadoop in order to solve some specific problems. So, let’s now talk about each one of these.
HDFS
Starting from the base of the Hadoop ecosystem, there is HDFS or Hadoop Distributed File System. It is a system that allows you to distribute the storage of big data across a cluster of computers. That means, all of your hard drives look like a single giant cluster on your system. That’s not all; it also maintains the redundant copies of data. So, if one of your computers happen to randomly burst into flames or if some technical issues occur, HDFS can actually recover from that by creating a backup from a copy of the data that it had saved automatically, and you won’t even know if anything happened. So, that’s the power of HDFS, i.e., the data storage is in a distributed manner having redundant copies.
YARN
Next in the Hadoop ecosystem is YARN (Yet Another Resource Negotiator). It is the place where the data processing of Hadoop comes into play. YARN is a system that manages the resources on your computing cluster. It is the one that decides who gets to run the tasks, when and what nodes are available for extra work, and which nodes are not available to do so. So, it’s like the heartbeat of Hadoop that keeps your cluster going.
MapReduce
One interesting application that can be built on top of YARN is MapReduce. MapReduce, the next component of the Hadoop ecosystem, is just a programming model that allows you to process your data across an entire cluster. It basically consists of Mappers and Reducers that are different scripts, which you might write, or different functions you might use when writing a MapReduce program. Mappers have the ability to transform your data in parallel across your computing cluster in a very efficient manner; whereas, Reducers are responsible for aggregating your data together. This may sound like a simple model, but MapReduce is very versatile. Mappers and Reducers put together can be used to solve complex problems. We will talk about MapReduce in one of the upcoming sections of this Hadoop tutorial.
Apache Pig
Next up in the Hadoop ecosystem, we have a technology called Apache Pig. It is just a high-level scripting language that sits on top of MapReduce. If you don’t want to write Java or Python MapReduce codes and are more familiar with a scripting language that has somewhat SQL-style syntax, Pig is for you.
It is a very high-level programming API that allows you to write simple scripts. You can get complex answers without actually writing Java code in the process. Pig Latin will transform that script into something that will run on MapReduce. So, in simpler terms, instead of writing your code in Java for MapReduce, you can go ahead and write your code in Pig Latin which is similar to SQL. By doing so, you won’t have to perform MapReduce jobs. Rather, just writing a Pig Latin code will perform MapReduce functions.
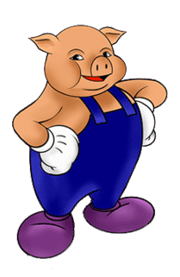
Hive
Now, in the Hadoop ecosystem, there comes Hive. It also sits on top of MapReduce and solves a similar type of problem like Pig, but it looks more like a SQL.
So, Hive is a way of taking SQL queries and making the distributed data sitting on your file system somewhere look like a SQL database. It has a language known as Hive SQL. It is just a database in which you can connect to a shell client and ODBC (Open Database Connectivity) and execute SQL queries on the data that is stored on your Hadoop cluster even though it’s not really a relational database under the hood. If you’re familiar with SQL, Hive might be a very useful API or interface for you to use.
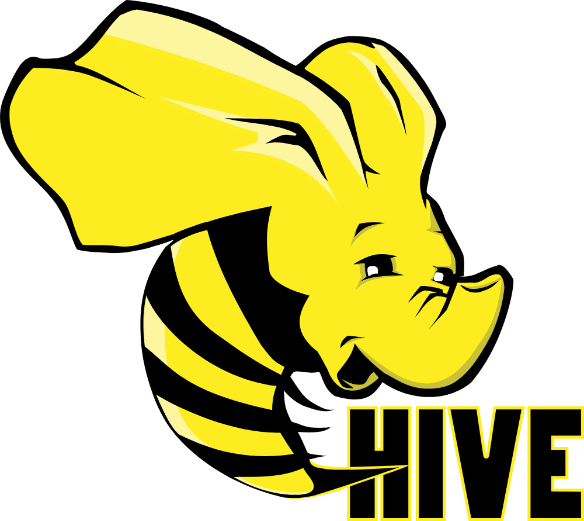
Apache Ambari
Apache Ambari is the next in the Hadoop ecosystem which sits on top of everything and gives you a view of your cluster. It is basically an open-source administration tool responsible for tracking applications and keeping their status.
It lets you visualize what runs on your cluster, what systems you’re using, and how much resources are being used. So, Ambari lets you have a view into the actual state of your cluster in terms of the applications that are running on it. It can be considered as a management tool that will manage the monitors along with the health of several Hadoop clusters.
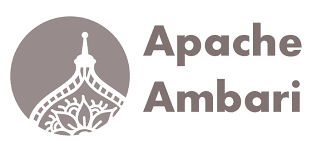
Mesos
Mesos isn’t really a part of Hadoop, but it’s included in the Hadoop ecosystem as it is an alternative to YARN. It is also a resource negotiator just like YARN.
Mesos and YARN solve the same problem in different ways. The main difference between Mesos and YARN is in their scheduler. In Mesos, when a job comes in, a job request is sent to the Mesos master, and what Mesos does is it determines the resources that are available and it makes offers back. These offers can be accepted or rejected. So, Mesos is another way of managing your resources in the cluster.
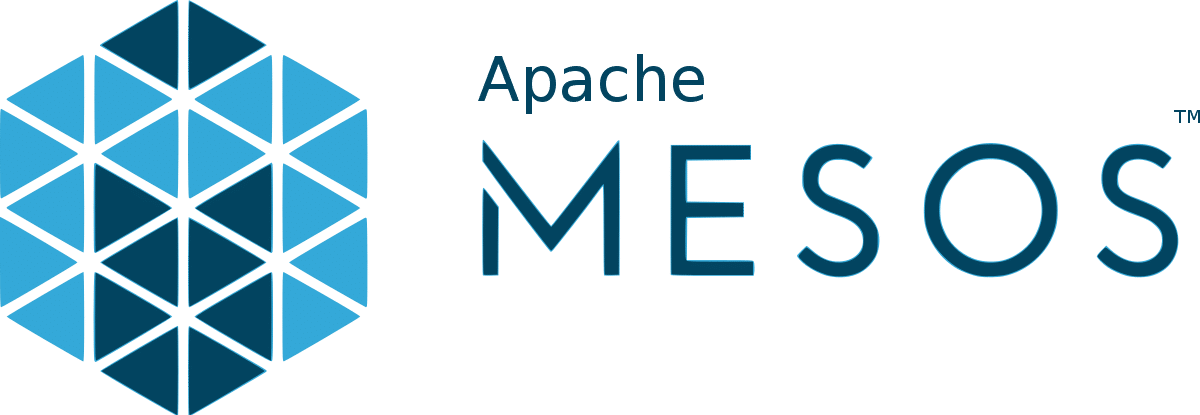
Apache Spark
Spark is the most interesting technology of this Hadoop ecosystem. It sits on the same level as MapReduce and right above Mesos to run queries on your data. It is mainly a real-time data processing engine developed in order to provide faster and easy-to-use analytics than MapReduce.
Spark is extremely fast and is under a lot of active development. It is a very powerful technology as it uses the in-memory processing of data. If you want to efficiently and reliably process your data on the Hadoop cluster, you can use Spark for that. It can handle SQL queries, do Machine Learning across an entire cluster of information, handle streaming data, etc.
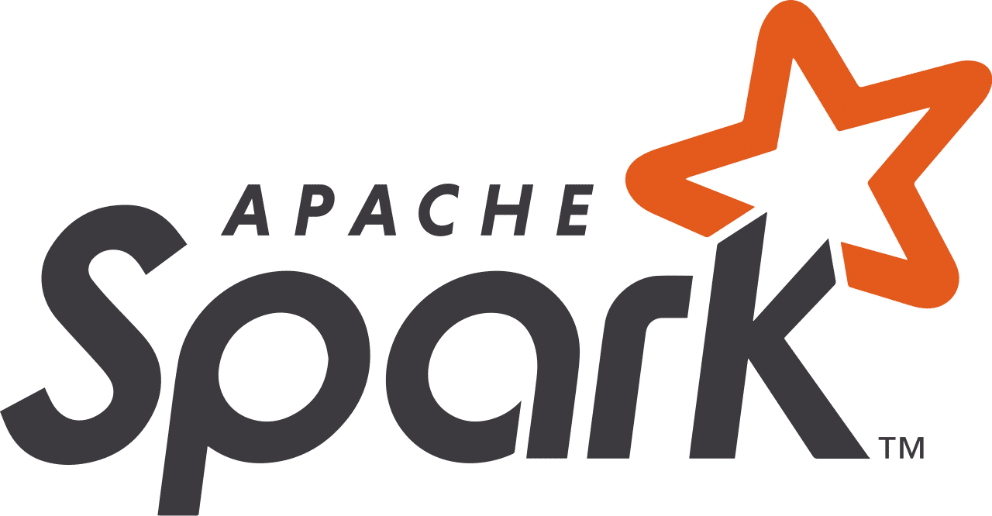
Tez
Tez is similar to Spark and is next in the Hadoop ecosystem it uses some of the same techniques as Spark. It tells you what MapReduce does as it produces a more optimal plan for executing your queries.
Tez, when used in conjunction with Hive, tends to accelerate Hive’s performance. Hive is placed on top of MapReduce, but you can place it on top of Tez, as Hive through Tez can be a lot faster than Hive through MapReduce. They are both different means of optimizing queries together.
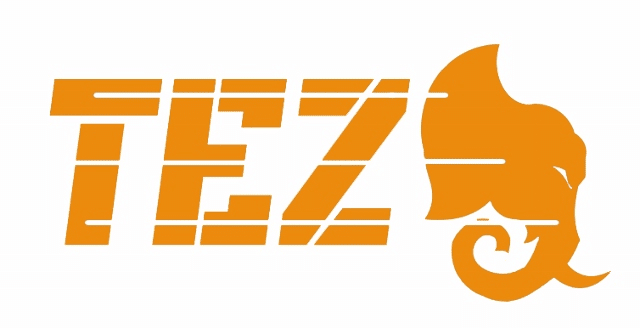
Apache HBase
Next up in the Hadoop ecosystem is HBase. It is set on the side, and it is a way of exposing data on your cluster to the transactional platform.
So, it is called the NoSQL database, i.e., it is a columnar data store that is a very fast database and is meant for large transaction rates. It can expose data stored in your cluster which might be transformed in some way by Spark or MapReduce. It provides a very fast way of exposing those results to other systems.
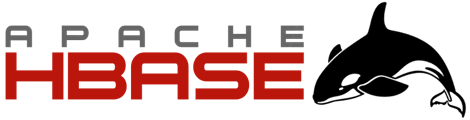
Apache Storm
Apache Storm is basically a way of processing streaming data. So, if you have streaming data from sensors or weblogs, you can actually process it in real time using Storm.
Processing data doesn’t have to be a batch thing anymore; you can update your Machine Learning models or transform data into the database, all in real time, as the data comes in.
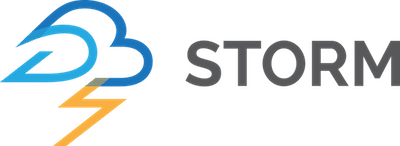
Oozie
Next up in the Hadoop ecosystem, there Oozie. Oozie is just a way of scheduling jobs on your cluster. So, if you have a task that needs to be performed on your Hadoop cluster involving different steps and maybe different systems, Oozie is the way for scheduling all these things together into jobs that can be run on some order.
So, when you have more complicated operations that require loading data into Hive, integrating that with Pig, and maybe querying it with Spark, and then transforming the results into HBase, Oozie can manage all that for you and make sure that it runs reliably on a consistent basis.
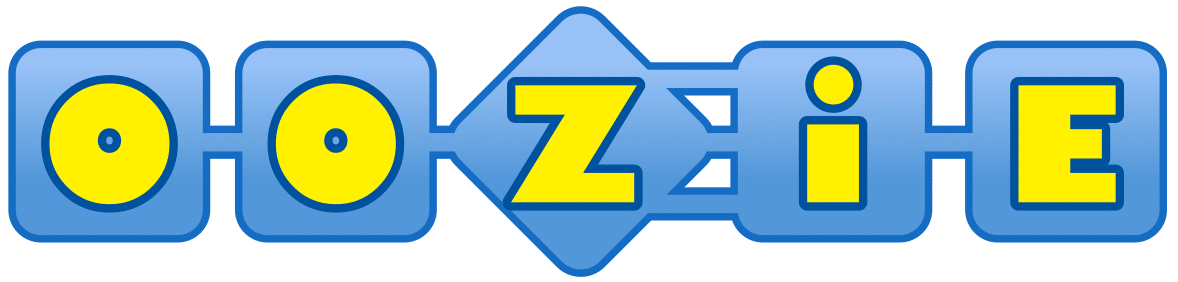
ZooKeeper
ZooKeeper is basically a technology for coordinating everything on your cluster. So, it is a technology that can be used for keeping track of the nodes that are up and the ones that are down.
It is a very reliable way of keeping track of shared states across your cluster that different applications can use. Many of these applications rely on ZooKeeper to maintain reliable and consistent performance across a cluster even when a node randomly goes down. Therefore, ZooKeeper can be used for keeping track of which the master node is, which node is up, or which node is down. Actually, it’s even more extensible than that.
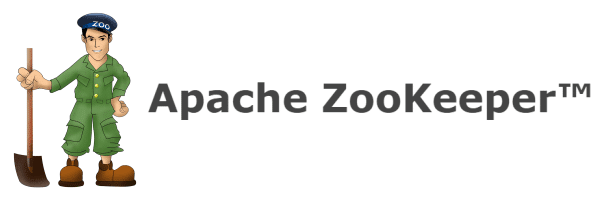
Data Ingestion
The below-listed systems in the Hadoop ecosystem are focused mainly on the problem of data ingestion, i.e., how to get data into your cluster and into HDFS from external sources. Let’s have a look at them.
- Sqoop: Sqoop is a tool used for transferring data between relational database servers and Hadoop. Sqoop is used to import data from various relational databases like Oracle to Hadoop HDFS, MySQL, etc. and to export from HDFS to relational databases.
- Flume: Flume is a service for aggregating, collecting, and moving large amounts of log data. Flume has a flexible and simple architecture that is based on streaming data flows. Its architecture is robust and fault-tolerant with reliable and recovery mechanisms. It uses the extensible data model that allows for online analytic applications. Flume is used to move the log data generated by application servers into HDFS at a higher speed.
- Kafka: Kafka is also an open-source streaming data processing software that solves a similar problem as Flume. It is used for building real-time data pipelines and streaming apps reducing complexity. It is horizontally scalable and fault-tolerant. Kafka aims to provide a unified, low-latency platform to handle real-time data feeds. Asynchronous communication and messages can be established with the help of Kafka. This ensures reliable communication.
In this section of the Hadoop tutorial, we learned about different Hadoop ecosystem components. We have so far learned 16 Hadoop components in the Hadoop ecosystem. In the next section of this tutorial, we will be learning about HDFS in detail.
Honestly thrilled with my choice to go for a static site supported by the INK for ALL app's Hugo-compatible export capability
ReplyDelete